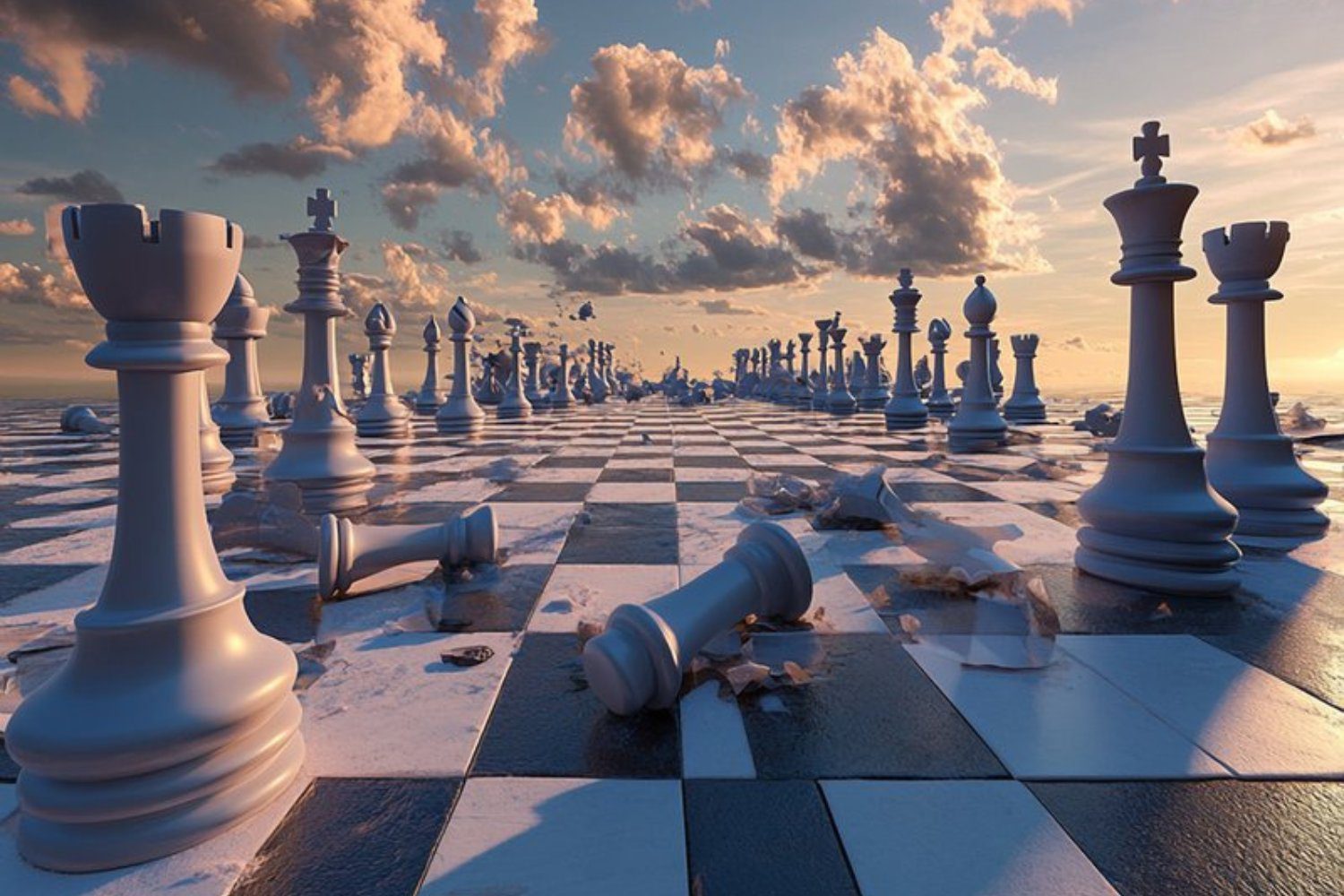
A team of researchers has pioneered an innovative artificial intelligence system that offers a radically different approach to problem-solving. Rather than focusing on immediate results, this AI can strategize across a vast number of possibilities, considering millions of steps ahead.
Led by mathematician Sergei Gukov at the California Institute of Technology (Caltech), the team developed a cutting-edge machine-learning algorithm specifically tailored to tackle intricate mathematical challenges that require an exceptionally lengthy series of operations—sometimes exceeding a million steps.
One notable accomplishment of this AI is its progress on the challenging Andrews–Curtis conjecture, a long-standing puzzle that has left mathematicians perplexed for many years. The conjecture fundamentally asks whether certain mathematical problems can always be solved through specific permitted operations, like rearranging or reversing steps.
According to Ali Shehper, the study’s lead author and a mathematician at Rutgers University, the aim of the new program was to discover long sequences of steps that are both rare and challenging to identify. “Navigating this complex challenge is like wandering through an immense maze, where only one route leads to success,” she explained in a Caltech press release.
In their preprint published on arXiv last August and subsequently updated, Shehper and her collaborators elucidated how the newly developed AI adeptly addressed various issues related to the Andrews–Curtis conjecture, which delves into concepts of abstract algebra. It’s worth noting that while they did not directly resolve the conjecture itself, the researchers successfully debunked several potential counterexamples. Although disproving these counterexamples does not confirm the conjecture’s truth, it certainly strengthens its credibility.
“Eliminating certain counterexamples enhances our confidence in the original conjecture and enriches our understanding of the central issue,” Shehper noted. Gukov likened the intricate math problems to solving a Rubik’s Cube.
“Imagine you have a scrambled yet intricate Rubik’s Cube—your task is to restore it to its original configuration by experimenting with extensive sequences of moves, and you won’t recognize if you’re progressing toward a solution until the very last moment,” he clarified.
So, what enables the AI to achieve such feats? Its success lies in its innovative thinking. Employing a reinforcement learning strategy, the researchers initially introduced the AI to simpler math problems before gradually escalating the difficulty level. “It experiments with various maneuvers, receiving rewards for correctly solved problems,” Shehper detailed. “We emphasize extending successful strategies while nurturing an element of curiosity. Ultimately, the AI learns to devise new methods that surpass human capabilities. This is the essence of reinforcement learning.”
Through this process, the algorithm discovered how to initiate extensive and unconventional sequences of moves, referred to as “super moves.” In contrast, the responses generated by ChatGPT tend to be more conventional.
“For instance, if you request ChatGPT to compose a letter, it typically produces standard content. It’s rare to see anything particularly unique or innovative; it serves more as an echo,” Gukov remarked. “Our program excels at generating outlier responses.”
One significant application that could benefit from such predictive intelligence is financial forecasting. Although current machine learning technologies haven’t yet reached that level of sophistication, the researchers believe their techniques might someday contribute to advanced forecasting capabilities.
“Our program essentially identifies how to learn to learn,” Gukov explained. “It’s about thinking innovatively.” He emphasized that the team made considerable strides in a field of mathematics that has been stagnant for decades. Furthermore, Gukov and his colleagues have adopted methods that do not rely on extensive computing resources, ensuring that their findings are accessible to other researchers with limited computational capabilities.
While the direct implications of this research may not be immediately apparent in everyday life, it contributes to a broader initiative among scientists optimizing machine-learning algorithms to tackle global challenges for the benefit of humanity.
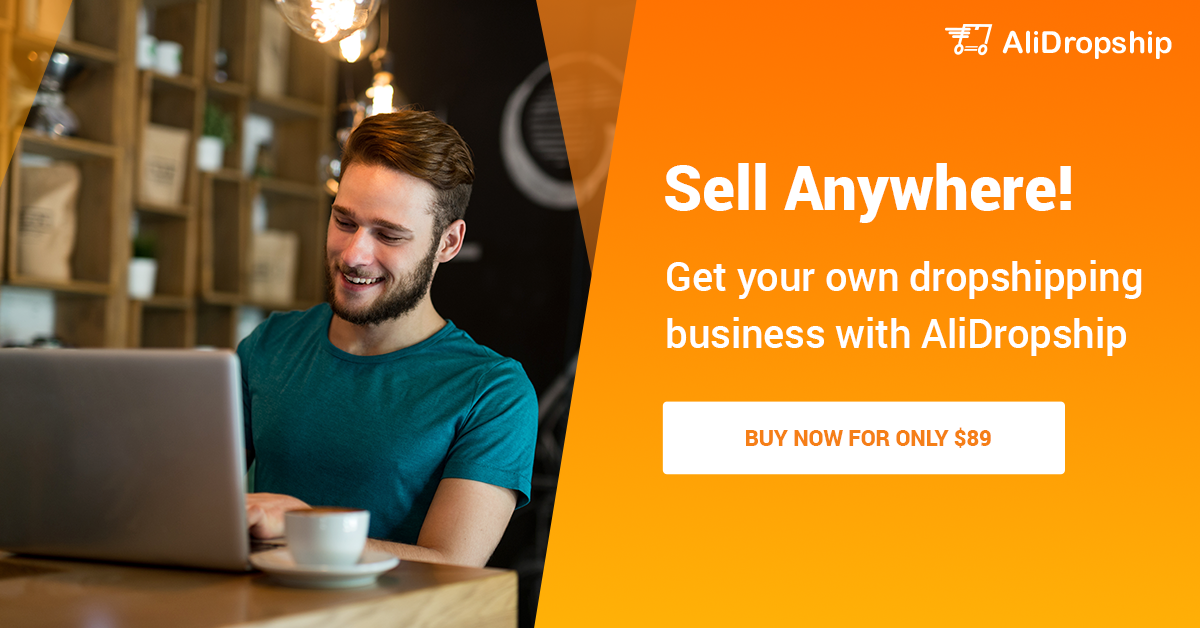