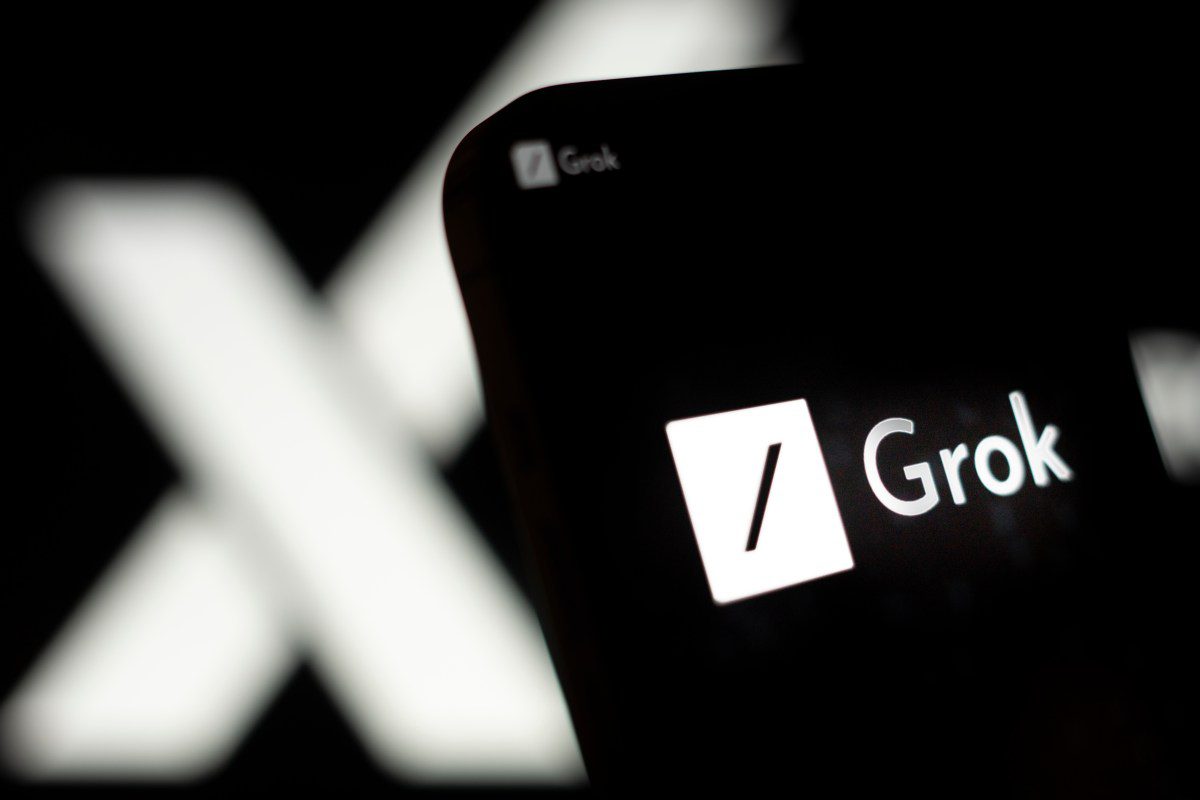
Ongoing discussions surrounding AI benchmarks and their reporting by laboratories have entered the public discourse.
Recently, an employee from OpenAI criticized xAI, the AI firm founded by Elon Musk, for allegedly sharing deceptive benchmark data related to its latest model, Grok 3. In response, xAI co-founder Igor Babushkin defended the company’s practices.
The reality seems to be more nuanced.
In a blog post by xAI, the firm showcased a graph illustrating Grok 3’s results on the AIME 2025 exam, which comprises a series of difficult math problems from a recent invitational competition. Some industry experts have raised concerns about the validity of AIME as a benchmark for AI performance. Nevertheless, both AIME 2025 and its predecessors are frequently utilized to assess a model’s mathematical capabilities.
According to the graph presented by xAI, Grok 3 Reasoning Beta and Grok 3 mini Reasoning outperformed OpenAI’s leading model, o3-mini-high, on the AIME 2025 assessments. However, OpenAI representatives on X quickly highlighted that xAI’s visual did not account for o3-mini-high’s score at “cons@64.”
What exactly does cons@64 stand for? It is shorthand for “consensus@64,” a method that allows a model 64 attempts to solve each problem in a benchmark, with the most frequently generated answers being chosen as the final responses. This approach can significantly enhance a model’s benchmark performance, and leaving it out of a graph may misleadingly imply that one model outperforms another when that isn’t entirely accurate.
When assessing Grok 3 Reasoning Beta and Grok 3 mini Reasoning’s AIME 2025 scores at “@1″—indicating the first attempt each model made—the results actually fell short of o3-mini-high’s score. Additionally, Grok 3 Reasoning Beta’s performance is only slightly behind OpenAI’s o1 model set to “medium” computing. Yet, xAI continues to promote Grok 3 as the “smartest AI in the world.”
Babushkin suggested on X that OpenAI has previously released similarly misleading benchmark graphics, although these focused on comparisons within its own models. A more impartial observer in this debate compiled a more “accurate” graph reflecting the performance of various models at cons@64:
It’s amusing how some interpret my plot as a critique against OpenAI while others see it as an attack on Grok, when in fact it serves as DeepSeek propaganda.
(I genuinely believe Grok looks strong here, and OpenAI’s dubious methods behind o3-mini-*high*-pass@”””1″” deserve further examination.) https://t.co/dJqlJpcJh8 pic.twitter.com/3WH8FOUfic— Teortaxes▶️ (DeepSeek 🐋铁粉 2023 – ∞) (@teortaxesTex) February 20, 2025
However, AI researcher Nathan Lambert pointed out in a post that perhaps the most critical metric is still elusive: the computational and financial resources each model utilized to achieve its peak performance. This indicates that many AI benchmarks fail to convey the true limitations and strengths of these models.
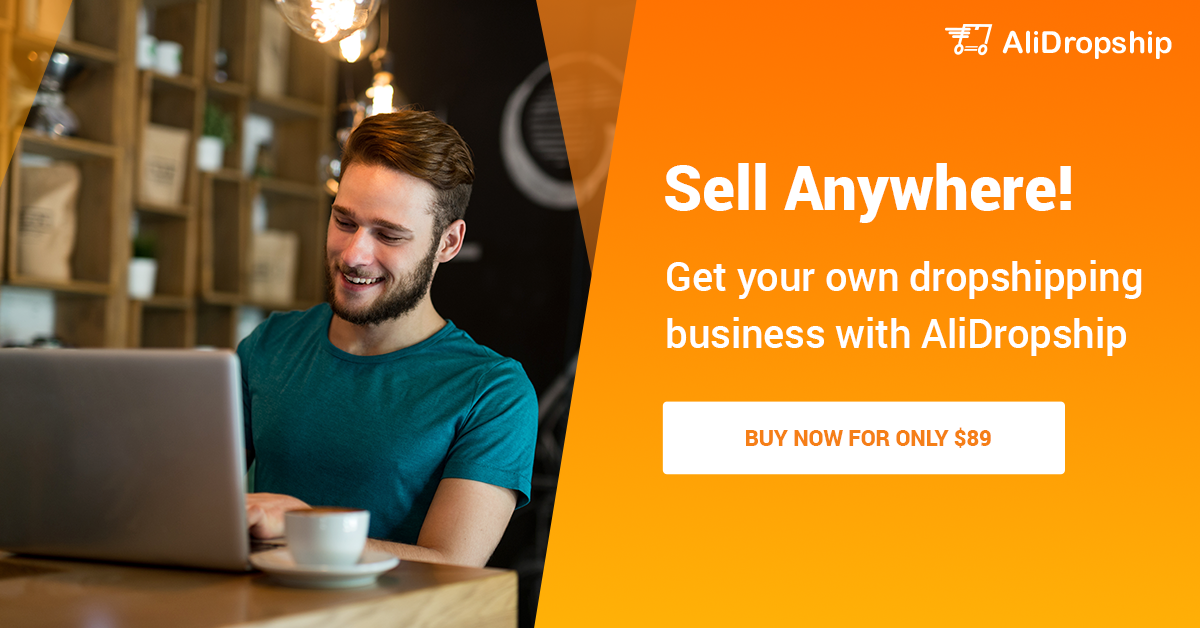